Every so often, I come across a story that I think would work well for this audience, only to find that it is actually just too light to justify a full article. Never one to deny you informative (and interesting) content, I’ve decided to alternate my usual long writing format with the occasional collection of short stories, tied together by a central thread but otherwise distinct from each other.
In v.01 of my Short Stories, I’m sharing five tales of AI to educate, captivate and horrify. Read them at your own peril.
The new world is (still) being built on African shoulders
When you picture the people who built ChatGPT, you probably envision a slick office in Silicon Valley, staffed with bespectacled tech virtuosos who spend their days and nights writing endless lines of code. The last thing that crosses your mind when you’re creating this image (I’m guessing) would be a building full of underpaid Kenyan data labellers.
For all its promise of changing the world as we know it back in 2022, the earliest versions of ChatGPT presented a significant stumbling block: its tendency to spit out racist, biassed and sexually suggestive answers. It makes sense if you consider that this is a large language model that was trained on that which already exists on the internet – and we all know what kind of stuff can be found on the web. From smut fiction to hate speech, this dicey content was poisoning the data pool and resulting in some seriously toxic responses.
The solution? To build a filter that would catch these kinds of responses before they made it past ChatGPT’s blinking cursor. Of course the building of this filter would require more human input than the usual data scraping that was used to train ChatGPT, because human beings would need to assess whether or not something was offensive. By identifying and labelling enough offensive inputs, they could feed ChatGPT a “guidebook” of topics, words and ideals that are not OK to use.
That’s where the Kenyans come in. In 2021, OpenAI (ChatGPT’s parent company) partnered with Sama, a data labelling partner based in San Francisco that claims to provide developing countries with “ethical” and “dignified digital work”. Sama recruited data labellers in Kenya to work on behalf of OpenAI, playing an essential role in making the chatbot safe for public usage. But despite their integral role in building ChatGPT, these workers faced gruelling conditions and low pay.
In order to obtain the labels it needed, OpenAI outsourced tens of thousands of text snippets to Sama, who then sent it to their Kenyan teams. Many of these texts seemed to originate from the darkest reaches of the internet, detailing graphic situations such as child sexual abuse, bestiality, murder, suicide, torture, self-harm and incest. Kenyan workers were expected to read and label between 150 and 250 passages of this text per nine-hour shift, with those passages ranging from around 100 words to well over 1,000. For this, they were paid a take-home wage of between around $1.32 and $2 per hour.
While the nature of this work eventually led to the cancellation of the contract between OpenAI and Sama, the harsh realities faced by these data labellers sheds light on a darker facet of the AI landscape. Beneath its techy allure lies a reliance on covert human labour in the Global South, often characterised by exploitation and harm. These unseen workers persist on the fringes, yet their contributions underpin billion-dollar industries.
Your dead granny wants you to sign up for premium
The digital world is brimming with personal remnants, from forgotten MySpace profiles to dormant social media pages, lingering online even after a person’s passing. But what if AI used these artefacts to recreate the presence of those we’ve lost?
It’s a reality unfolding before us as we speak – and one that AI ethicists caution could lead to a new phenomenon: “digital hauntings” by “deadbots.” With the ongoing advancements in generative artificial intelligence, there emerges a novel possibility for grieving individuals to interact with chatbot avatars trained on the digital footprint of the deceased, encompassing their voice, appearance and online persona.
Certain products from companies like Replika, HereAfter and Persona, marketed as “digital replicas”, are already pushing these boundaries, allowing users to simulate the departed. Amazon’s 2022 demonstration, wherein its Alexa assistant mimicked the voice of a deceased woman using just a brief audio clip, underscores the potential of this unsettling trend.
In their recent publication in Philosophy and Technology, AI ethicists Tomasz Hollanek and Katarzyna Nowaczyk-Basińska employed a technique known as “design fiction” to envisage various situations where fictional characters encounter challenges with different “postmortem presence” enterprises. The scenario that stuck with me the most is one where an adult user is impressed by the realism of their deceased grandparent’s chatbot, only to soon start receiving premium trial and food delivery service advertisements in their deceased relative’s voice.
“These services run the risk of causing huge distress to people if they are subjected to unwanted digital hauntings from alarmingly accurate AI recreations of those they have lost,” Hollanek added. “The potential psychological effect, particularly at an already difficult time, could be devastating.”
“We need to start thinking now about how we mitigate the social and psychological risks of digital immortality,” Nowaczyk-Basińska added.
No, that’s not really Katy Perry
Earlier this month, New York City saw a grand gathering of music, entertainment and fashion icons for the annual Met Gala, themed “Garden of Time”.
However, amidst the glamorous snapshots flooding social media, Katy Perry was notably absent. Or was she?
Despite the fact that Perry was in studio recording while the Met celebrations went on, two images of her (wearing two completely different dresses) seemingly posing for photographers at the Met started circulating on social media channels as other celebrities made their entrances.
While it’s unclear where these AI-generated images originated from, they were realistic enough that they were shared and liked thousands of times before sharp-eyed viewers cried foul. According to a screenshot shared by Perry herself, even her mother was fooled into commenting on her flowered ball gown.
No, that’s not really Scarlett Johansson
Less amused by AI’s imitation of her is Scarlett Johansson, according to recent headlines.
The actress stated that she was left “shocked and angered” after OpenAI launched a chatbot this month with a voice that sounds “eerily similar” to hers.
This comes on the heels of Johansson turning down an offer from OpenAI to voice the chatbot, named Sky, in September last year. Two days before Sky went live, Sam Altman reached out to Johansson again, requesting that she rethink the offer. She declined, and the contested demo version of the chatbot went live days later.
In a statement shared with the BBC by OpenAI, Mr Altman denied that the company had sought to imitate Johansson’s voice. “The voice is not Scarlett Johansson’s, and it was never intended to resemble hers,” he wrote.
Johansson’s lawyers have retaliated by sending two letters to OpenAI, demanding insights into how the voice was created. While OpenAI continues to deny that the voice of its chatbot was designed to imitate Johansson, they have since suspended the use of that particular voice.
The AI train is running out of coal
As AI continues to soar in popularity, researchers have raised concerns that the industry could be facing a shortage of training data – the essential fuel powering advanced AI systems. This potential scarcity threatens to decelerate the development of AI models, particularly the expansive language models that rely heavily on vast datasets.
In other words: after scouring the world wide web for half a decade and scraping data from billions of sites (presumably both legally and slightly-less-than-legally), AI is running out of fresh data to learn from.
When I first read this headline, I was a bit surprised that we could be at this junction already. To me it feels as though OpenAI and its cousins have only really been a major part of our lives since 2022. Consider that the internet itself is over four decades old – that’s four decades worth of writing. It’s a bit astonishing to consider that these large language models have burned through this much fuel already.
Obviously, training powerful, accurate and high-quality AI algorithms requires an immense amount of data. For example, ChatGPT was trained on an extensive dataset encompassing 570 gigabytes of text data, equivalent to roughly 300 billion words.
In a similar vein, the stable diffusion algorithm, which powers many AI image-generating applications like DALL-E, Lensa and Midjourney, was trained on the LIAON-5B dataset. This dataset includes an impressive 5.8 billion image-text pairs.
High-quality AI models cannot be developed using low-quality data sources, such as social media posts or blurry photographs, despite their abundance and ease of access. These types of data do not provide the richness and precision needed to train high-performing AI systems. Therefore, ensuring both the quantity and quality of data is paramount for the advancement and reliability of AI technologies.
To further complicate the problem (although this writer in particular was quite gleeful to learn this fact), AI models cannot be trained on AI-generated content. All of those blogs and LinkedIn articles that are being churned out at pace by ChatGPT? Those are absolutely useless for training purposes – in fact, their inclusion in learning datasets can actually cause damage to the algorithm. By making ChatGPT so freely available to the masses, OpenAI has effectively contributed to its own training data shortage.
(For the record, I don’t usually subscribe to schadenfreude. But when something has messed with your industry and your ability to work as seemingly effortlessly as ChatGPT has, well… it does make you smile a little to see them struggle for once.)
So, what next? Innovation, of course. The people building AI models are way too smart to be held back by something as trivial as a data shortage. One promising avenue for AI developers is to enhance algorithms to utilise existing data more efficiently. By refining these algorithms, it is likely that, in the coming years, they will be able to train high-performing AI systems using less data and potentially less computational power. This optimisation would not only advance the capabilities of AI but also contribute to reducing its carbon footprint, addressing environmental concerns associated with large-scale data processing.
Another viable strategy is the use of specially-designed AI to generate synthetic data for training systems. In this approach, developers can create the specific data they require, tailored precisely to the needs of their particular AI model. Obviously, this kind of content differs from the break-the-algorithm type of AI-generated content I mentioned before. This synthetic data can mimic real-world data scenarios without the need for massive data collection efforts. It can fill gaps in datasets, provide diversity in training examples, and even help in creating rare or hard-to-obtain data instances.
By focusing on these strategies, the AI industry can overcome the challenges posed by data limitations, paving the way for more sustainable and efficient AI advancements.
Hooray for that, I guess. Or not.
About the author: Dominique Olivier, founder human.writer
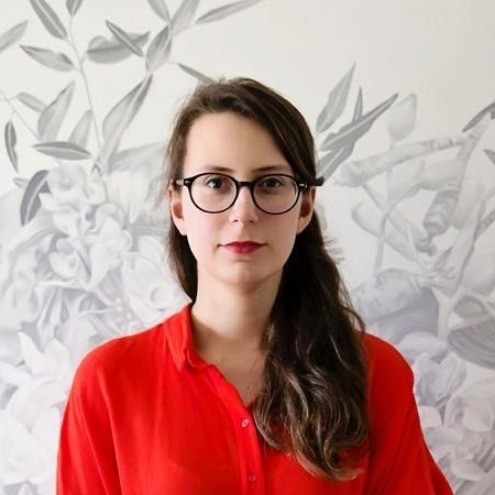
Dominique Olivier is the founder of human.writer, where she uses her love of storytelling and ideation to help brands solve problems.
She is a weekly columnist in Ghost Mail and collaborates with The Finance Ghost on Ghost Mail Weekender, a Sunday publication designed to help you be more interesting.
Dominique can be reached on LinkedIn here.